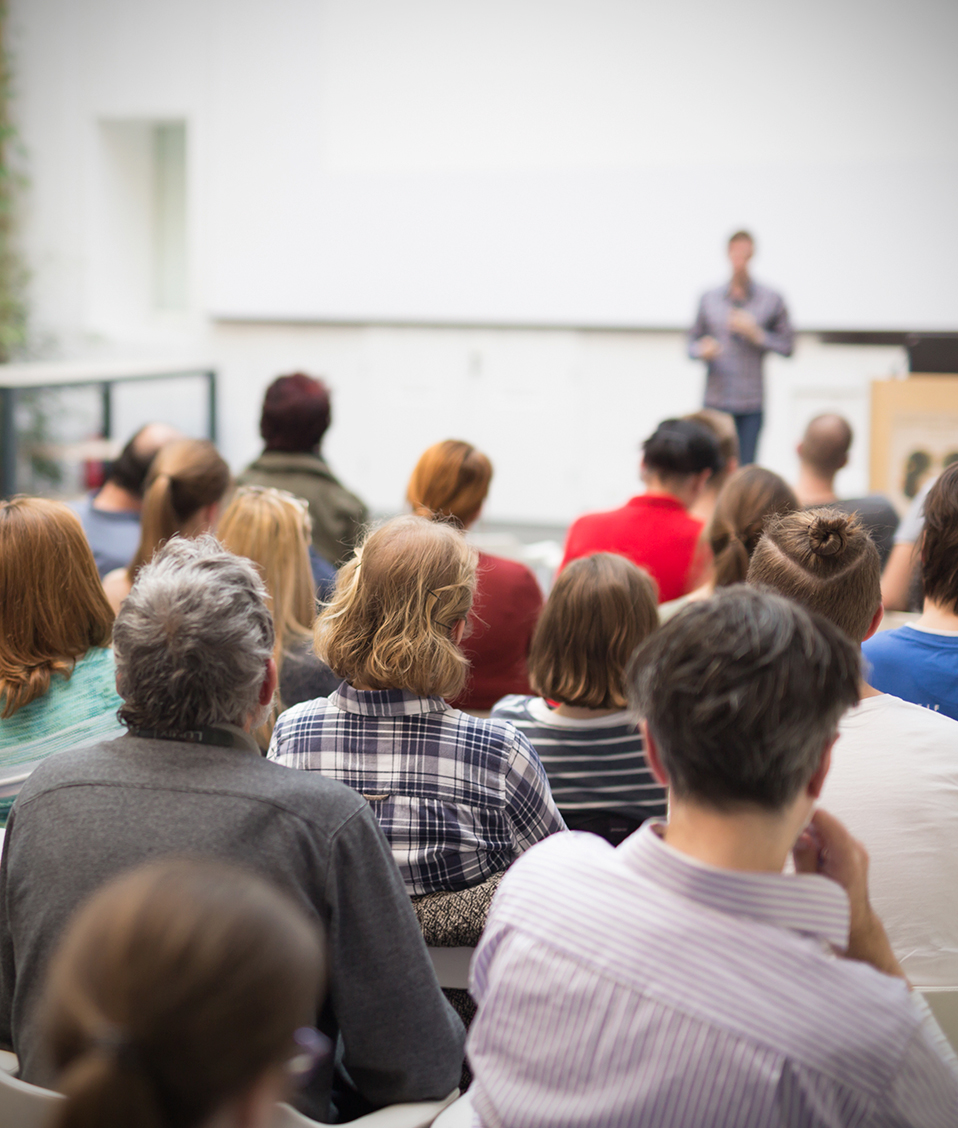
Title: Measuring the informativeness of decision-making models
Room: E28
Time: 12:30
Abstract: Experimental evidence of systematic deviations from rationality have inspired numerous alternative models of decision-making. Choosing among these models is a non-trivial task, especially since they often do not explicitly account for errors (i.e. they are deterministic) and have varying degrees of flexibility. This paper presents a new measure that can be used to assess the informativeness of different models with respect to a given dataset. Our measure trades off model fit and flexibility in a parsimonious way, allowing for straightforward comparison of non-nested models. We use this measure to evaluate deterministic choice models and infer subjects' preferences in classic decision-making experiments. We show how this measure allows for the recovery of choice correspondences (for example by recovering indifferences) and appears to perform well out of sample.